LLMs: Transforming Data Analysis - From Sidekick to Partner
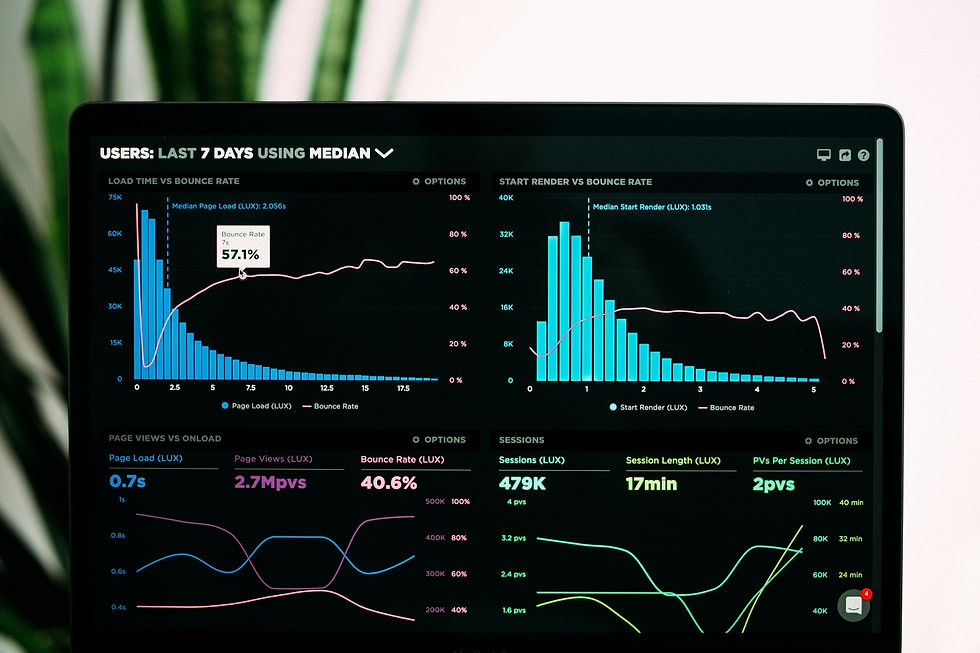
Basic understanding of LLMs
Large language models (LLM) are very large deep learning models that are pre-trained on vast amounts of data. The underlying transformer is a set of neural networks that consist of an encoder and a decoder with self-attention capabilities. The encoder and decoder extract meanings from a sequence of text and understand the relationships between words and phrases in it.
Note: A neural network is a method in artificial intelligence that teaches computers to process data in a way that is inspired by the human brain. It is a type of machine learning process, called deep learning, that uses interconnected nodes or neurons in a layered structure that resembles the human brain. It creates an adaptive system that computers use to learn from their mistakes and improve continuously. Thus, artificial neural networks attempt to solve complicated problems, like summarizing documents or recognizing faces, with greater accuracy.
Large language models are incredibly flexible. One model can perform completely different tasks such as answering questions, summarizing documents, translating languages, and completing sentences. LLMs have the potential to disrupt content creation and the way people use search engines and virtual assistants.
How do LLMs work?
A key factor in how LLMs work is the way they represent words. Earlier forms of machine learning used a numerical table to represent each word. But, this form of representation could not recognize relationships between words such as words with similar meanings. This limitation was overcome by using multi-dimensional vectors, commonly referred to as word embeddings, to represent words so that words with similar contextual meanings or other relationships are close to each other in the vector space.
Basic understanding of Data Analytics
Data analytics refers to the process of examining and interpreting large volumes of data to uncover meaningful insights, patterns, and trends. It involves applying statistical and analytical techniques to extract valuable information from raw data.
Data analytics aims to gain a deeper understanding of the data and use that knowledge to make informed decisions or drive improvements in various aspects of business operations.
The Future of LLMs and Data Analytics
As the field of data analytics continues to evolve, the integration of LLMs holds immense potential for future developments. LLMs and data analytics are expected to undergo several advancements to further enhance their capabilities and impact various industries.
Improved Language Understanding
Future advancements in LLM technology will likely focus on improving language understanding capabilities. LLMs will become more proficient in recognizing context, sarcasm, idioms, and other linguistic nuances.
Real-Time Analysis
The future of LLMs in data analytics will involve real-time analysis capabilities. LLMs will be able to process and analyze textual data in real-time, allowing businesses to respond swiftly to emerging trends, customer feedback, and market dynamics.
Integration with Other Data Analytics Techniques
Future developments will focus on integrating LLM-generated insights with other data analytics techniques. By combining LLMs with traditional statistical models, machine learning algorithms, and predictive analytics, businesses can harness each approach’s strengths and create more accurate predictive models.
Ethical and Bias Considerations
As LLMs’ usage in data analytics becomes more prevalent, there will be an increased focus on ethical considerations and addressing potential biases. Future developments will improve transparency and fairness in LLM algorithms, ensuring that they do not perpetuate biases or discriminate against certain groups.
Concerns about LLMs in Data Analytics
What’s the role for humans in analytics if AI takes over the job?
Some data professionals worry that these high-powered tools may undermine human expertise and intuition in complex problem-solving. After all, they do point to a new role for human judgment, creativity, and experience, raising concerns about problem-solving processes.
However, LLMs used in data analytics should be viewed as a complementary tool rather than a replacement for human expertise. These models are designed to assist and enhance decision-making by quickly processing vast amounts of information and identifying patterns that may elude human perception. Human intuition and expertise remain indispensable in interpreting results, contextualizing findings, and making nuanced decisions based on a deep understanding of complex situations. This approach can empower humans to make more informed and strategic decisions in collaboration with advanced computational tools.
Conclusion:
The entry of LLMs into the data analysis arena marks a turning point, not a takeover. As we've seen, they offer exciting prospects for increased efficiency, deeper text-based insights, and creative exploration. However, their limitations in transparency, data dependence, and the irreplaceable human element make collaboration, not competition, the key to success.
Imagine a future where analysts seamlessly integrate LLMs into their workflows, utilizing their strengths while maintaining critical thinking and ethical considerations. This symphony of human and machine intelligence will unlock unprecedented data understanding, empowering informed decision-making and shaping a brighter future for all.
Let's not simply use LLMs, but partner with them. Together, we can unlock the true power of data analysis and make the world a more informed and insightful place.
Reference links:
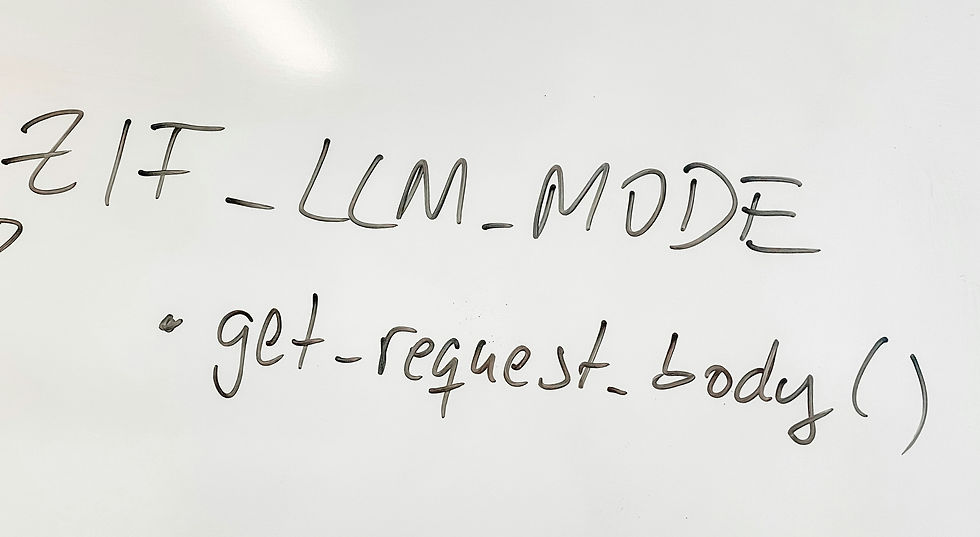